Can We Learn to Live with AI Hallucinations?
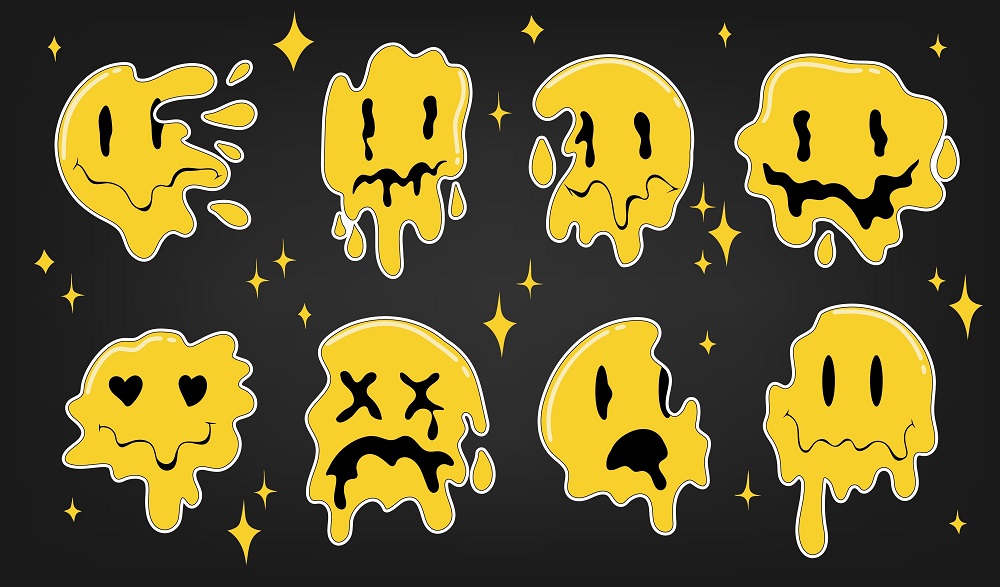
The generative AI revolution is remaking businesses’ relationship with computers and customers. Hundreds of billions of dollars are being invested in large language models (LLMs) and agentic AI, and trillions are at stake. But GenAI has a significant problem: The tendency of LLMs to hallucinate. The question is: Is this a fatal flaw, or can we work around it?
If you’ve worked much with LLMs, you have likely experienced an AI hallucination, or what some call a confabulation. AI models make things up for a variety of reasons: erroneous, incomplete, or biased training data; ambiguous prompts; lack of true understanding; context limitations; and a tendency to overgeneralize (overfitting the model).
Sometimes, LLMs hallucinate for no good reason. Vectara CEO Amr Awadallah says LLMs are subject to the limitations of data compression on text as expressed by the Shannon Information Theorem. Since LLMs compress text beyond a certain point (12.5%), they enter what’s called “lossy compression zone” and lose perfect recall.
That leads us to the inevitable conclusion that the tendency to fabricate isn’t a bug, but a feature, of these types of probabilistic systems. What do we do then?
Countering AI Hallucinations
Users have come up with various methods to control or for hallucinations, or at least to counteract some of their negative impacts.
For starters, you can get better data. AI models are only as good as the data they’re trained on. Many organizations have raised concerns about bias and the quality on their data. While there are no easy fixes to improving data quality, customers that dedicate resources to better data management and governance can make a difference.
Users can also improve the quality of LLM response by providing better prompts. The field of prompt engineering has emerged to serve this need. Users can also “ground” their LLM’s response by providing better context through retrieval-augmented generation (RAG) techniques.
Instead of using a general-purpose LLM, fine-tuning open source LLMs on smaller sets of domain- or industry-specific data can also improve accuracy within that domain or industry. Similarly, a new generation of reasoning models, such as DeepSeek-R1 and OpenAI o1, that are trained on smaller domain-specific data sets, include a feedback mechanism that allows the model to explore different ways to answer a question, the so-called “reasoning” steps.
Implementing guardrails is another technique. Some organizations use a second, specially crafted AI model to interpret the results of the primary LLM. When a hallucination is detected, it can tweak the input or the context until the results come back clean. Similarly, keeping a human in the loop to detect when an LLM is headed off the rails can also help avoid some of LLM’s worst fabrications.
AI Hallucination Rates
When ChatGPT first came out, its hallucination rate was around 15% to 20%. The good news is the hallucination rate appears to be going down.
For instance, Vectara’s Hallucination Leader Board uses the Hughes Hallucination Evaluation Model–which calculates the odds of an output being true or false on a range from 0 to 1. Vectara’s hallucination board currently shows several LLMs with hallucination rates below 1%, led by Google Gemini-2.0 Flash. That’s a big improvement form a year ago, when Vectara’s leaderboard showed the top LLMs had hallucination rates of around 3% to 5%.
Other hallucinations measures don’t show quite the same improvement. The research arm of AIMultiple benchmarked nine LLMs on the capability to recall information from CNN articles. The top-scoring LLM was GPT-4.5 preview with a 15% hallucination rate. Google’s Gemini-2.0 Flash at 60%.
“LLM hallucinations have far-reaching effects that go well beyond small errors,” AIMultiple’s Principal Analyst Cem Dilmegani wrote in a March 28 blog post. “Accurate information produced by an LLM could result in legal ramifications, especially in regulated sectors such as healthcare, finance, and legal services. Organizations could be penalized severely if hallucinations caused by generative AI lead to infractions or negative consequences.”
High-Stakes AI
One company working to make AI usable for some high-stakes use cases is the search company Pearl. The company combines an AI-powered search engine along with human expertise in professional services to minimize the odds that a hallucination will reach a user.
Pearl has taken steps to minimize the hallucination rate in its AI-powered search engine, which Pearl CEO Andy Kurtzig said is 22% more accurate than ChatGPT and Gemini out of the box. The company does that by using the standard techniques, including multiple models and guardrails. Beyond that, Pearl has contracted with 12,000 experts in fields like medicine, law, auto repair, and pet health who can provide a quick sanity check on AI-generated answers to further drive the accuracy rate up.
“So for example, if you have a legal issue or a medical issue or an issue with your pet, you’d start with the AI, get an AI answer through our superior quality system,” Kurtzig told BigDATAwire. “And then you’d get the ability to then have to get a verification from an expert in that field, and then you can even take it one step further and have a conversation with the expert.”
Kurtzig said there are three major unresolved problems around AI: the persistent problem of AI hallucinations; mounting reputational and financial risk; and failing business models.
“Our estimate on the state of the art is roughly a 37% hallucination level in the professional services categories,” Kurtzig said. “If your doctor was 63% right, you would be not only pissed, you’d be suing them for malpractice. That is awful.”
Big, rich AI companies are running a real financial risk by putting out AI models that are prone to hallucinating, Kurtzig said. He cites a Florida lawsuit filed by the parents of a 14-year-old boy who killed himself when an AI chatbot suggested it.
“When you’ve got a system that is hallucinating at any rate, and you’ve got really deep pockets on the other end of that equation…and people are using it and relying, and these LLMs are giving these highly confident answers, even when they’re completely wrong, you’re going to end up with lawsuits,” he said.
Diminishing AI Returns
The CEO of Anthropic recently made headlines when he claimed that 90% of coding work would be done by AI within months. Kurtzig, who employs 300 developers, doesn’t see that happening anytime soon. The real productivity gains are somewhere between 10% and 20%, he said.
The combination of reasoning models and AI agents is supposed to be heralding a new era of productivity, not to mention a 100x increase in inference workloads to occupy all those Nvidia GPUs, according to Nvidia CEO Jensen Huang. However, while reasoning models like DeepSeek can run more efficiently than Gemini or GPT-4.5, Kurtzig doesn’t see them increasing the state of the art.
“They’re hitting diminishing returns,” Kurtzig said. “So each new percentage of quality is really expensive. It’s a lot of GPUs. One data source I saw from Georgetown says to get another 10% improvement, it’s going to cost $1 trillion.”
Ultimately, AI may pay off, he said. But there’s going to be quite a bit of pain before we get to the other side.
“Those three fundamental problems are both huge and unsolved, and they are going to cause us to head into the trough of disillusionment in the hype cycle,” he said. “Quality is an issue, and we’re hitting diminishing returns. Risk is a huge issue that is just starting to emerge. There’s a real cost there in both human lives as well as money. And then these companies are not making money. Almost all of them are losing money hand over fist.
“We got we got a trough of disillusionment to get through,” he added. “There is a beautiful plateau of productivity out on the other end, but we haven’t hit the trough of disillusionment yet.”
Related Items:
What Are Reasoning Models and Why You Should Care
Vectara Spies RAG As Solution to LLM Fibs and Shannon Theorem Limitations
Hallucinations, Plagiarism, and ChatGPT
The post Can We Learn to Live with AI Hallucinations? appeared first on BigDATAwire.